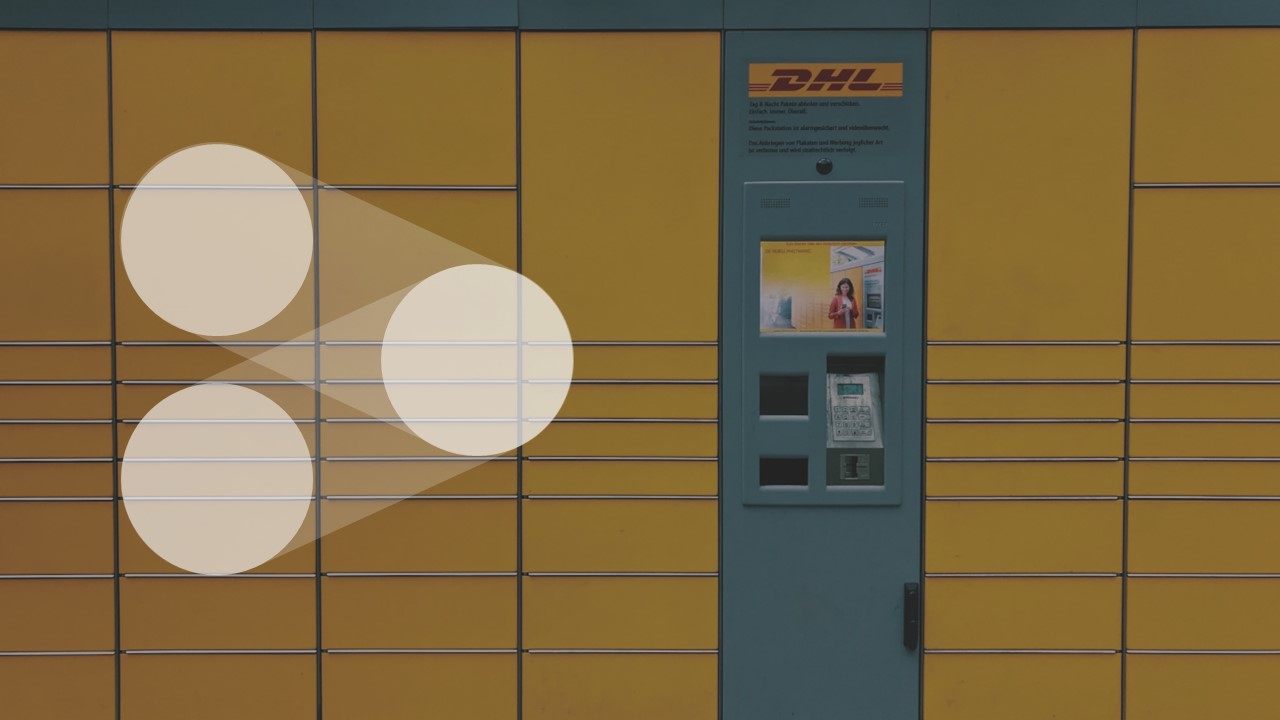
What is Self-Service Analytics & How to Implement it
by Katy Yuan, Marketing Manager
What is Self-Service Analytics & How to Implement it
by Katy Yuan, Marketing Manager
With quintillion of data generated every day, consuming data is both an opportunity and a source of frustration. Luckily, analytics can tame this data chaos. However, when analytics is restricted in the hands of a few technical experts, organizations cannot fully exploit the potential of their data. Self-service analytics opens avenues for business decision makers to access data easily and use actionable insights to help improve their decisions and work.
What is Self-Service Analytics?
Self-service analytics refers to the ability to analyze data, visualize insights, create dashboards, and generate reports all by oneself in a self-service manner, without depending on experts or undergoing extensive technical training. Everyone in the organization, from IT and data analysts to business executives and on-field teams, benefits from self-service analytics. The non-technical business users gain easy and quick access to insights for their daily decision making, whereas the IT teams and data analysts are freed from the time-consuming and iterative information requests and can focus better on complex problems and innovations.
Using self-service analytics , anyone can ask questions to their enterprise data in a simple language, without using complex queries or syntax. For example, sales managers can ask “Why did sales increase in 2021?” or customer success teams can ask “What was the customer churn last quarter?” or marketing executives can ask “Show me the most used coupon codes by transactions”. Self-service analytics not only provides instant answers but also helps users understand and interpret them better with visualizations and text summaries.
Who Should Use Self-Service Analytics?
Through self-service analytics tools, organizations can now empower every business user to become an augmented consumer of data insights. When organizations extend analytics capabilities to their users, they empower them with insights to reach their goals better. Research by Harvard Business Review Analytic Services establishes that organizations can substantially improve business performance by giving frontline workers modern self-service analytics tools to enable fast and intelligent actions.
Self-service analytics tools have found applications across industries and address use cases in various business functions including marketing, retail, customer success, or revenue operations. Users can interact with, discover, and consume insights on demand and at the point of decision-making. Thanks to artificial intelligence, insights are now more personalized and actionable than ever before, leading to optimized efficiency and costs for a data-literate organization.
Key Highlights of a Self-Service Analytics Platform
While evaluating self-service analytics platforms, here are some capabilities that you must consider.
1. Intuitive Search
This is the most fundamental capability of a self-service analytics platform. Is the search readily available, intuitive, powerful, and easy to use? Is the search intelligent enough to understand a user’s natural language and parse it to extract contextual answers? Users should be able to ask queries such as “product with highest sales this quarter” and get relevant answers instantly.
2. Decision Intelligence
Today, decision makers want to know beyond what happened, to understand why it happened, and how they can make better decisions. Self-service analytics platforms must provide such decision intelligence in the form of actionable insights, personalized recommendations, and real-time automated insights.
3. Interactive Visualizations
Gone are the days when decision makers would spend hours and hours peering into lengthy reports and static dashboards, trying to make sense of the numbers. Self-service analytics platforms must make insights more consumable by offering interactive visualizations, engaging audio-visual data stories, audio narrations, and text summaries.
4. Data Security and Governance
The importance of security and proper governance in data cannot be underlined enough in today’s highly connected digital world. Self-service analytics platforms must open access for users to interact with data, and at the same time, apply granular policies to ensure that the right people have access to the right data.
5. Embedded Analytics
When insights are embedded in a business workflow, users don’t have to switch between applications and can benefit from readily available insights at the point of decision-making. Self-service analytics platforms must offer embedded analytics capabilities such as embedded search, insights, dashboards, and visualizations to reduce friction, provide a seamless experience, and boost data-driven decision-making.
Benefits of Using Self-Service Analytics
Organizations across industries have been seeing the benefits of self-service analytics tools. Here are some of the most important benefits.
1. Greater Data Accessibility
Self-service analytics tools are a requirement for data literacy. Data democratization initiatives must provide a friendly interface for all users to consume insights easily, without requiring extensive training or technical knowledge. In order to successfully create a data literate workforce, users need tools that help them dive deep into data and generate deep insights that aid decision-making. Here, taking advantage of AI-Powered Analytics boosts the usability of data by augmenting decisions with personalized, timely insights.
2. Data Resource Optimization
By reducing dependency on IT, self-service analytics frees up valuable human resources, such as Data Scientists, to focus on larger projects that require manual effort. Their expertise can be put towards complex challenges such as revenue forecasting models, competitive intelligence, and predicting market trends. On the other hand, business users can quickly automate less complex activities, such as data visualization, exploration, and periodic reporting.
3. Greater Data Accuracy
Self-service analytics encourages a single source of truth as all consumers receive data from a centralized data source updated in real time. Today, ad hoc analysis is often done offline in Excel sheets or static reports, resulting in data silos and outdated insights. Self-service instant insights break through the data silos that may hamper accuracy.
4. Improved Decision-making
In addition to basing business decisions on live data, self-service analytics further improves data-driven decision-making by expediting data access. Business users no longer have to wait for input from IT or data analysts, and can immediately tap into personalized insights to gather actionable recommendations. Organizations can now become insight-driven, instead of simply data-driven.
5. Cost Efficiency
Advanced self-service analytics tools make businesses cost-efficient by saving manpower, streamlining access to data, and helping users make the right decisions at the right time. In addition, high user adoption means better scalability across the organization and faster time-to-insight for new employees. Many companies are also turning to modern cloud analytics for rapid onboarding and deployment across multiple business units.
Implementing Self-Service Analytics in Your Organization
1. Prioritize business workflows that require data-driven insights
Start by separating vanity metrics from the actual business metrics that are most important for your business. Prioritize the business workflows that are complex, time-consuming, recurrent, and need the latest data. Consider implementing self-service analytics for such processes to expedite actions, minimize risk, and reduce delays. Review the outcomes and apply the learnings from these workflows to further apply self-service analytics to other business workflows gradually.
2. Identify data sources for business workflows
Different departments use different stacks for collecting and storing data. Decisions made based on incomplete and outdated data can lead to incorrect actions and disastrous outcomes. Perform a thorough inventory of all structured data sources. This provides clear visibility of available data and uncovers silos for consolidating all data. Look for direct data connector capabilities in self-service analytics tools that offer better data integration and prevent data duplication.
3. Opt for low-prep/no-prep onboarding of high-quality data
Automating data processes can save significant time and efforts otherwise spent in manually cleaning and preparing data. Modern data analytics tools that offer automated data cataloging, entity relationship building, and metadata enrichment can ensure low-prep/no-prep onboarding. Once data is integrated, check for any missing or incomplete values, inconsistencies, and errors. If the data itself is incorrect, has duplicate or missing values, and is not consistent in nature, it loses its credibility to provide accurate insights. So verifying the quality of data is a must to get accurate insights.
4. Democratize access to data and insights
Don’t restrict data-driven decision-making abilities only to high-level executives. Consider enabling it for the larger frontline workforce too such as account associates, sales representatives, marketing teams, customer success teams, or retail store managers. This ensures better decisions and actions at all levels within an organization. Put in place clear data governance policies to ensure that the teams get access to relevant data based on their roles, work, or geography. This helps bring transparency and accountability while democratizing data and insights for everyone in the organization.
5. Provide better data exploration and visualization capabilities
Traditional analytics tools, with their complex syntax, SQL queries, and steep learning curves, make analytics feel like an overhead, especially for non-technical business users. When users are empowered with intuitive interfaces, search-based data querying, AI-based data visualizations, and audio-visual data stories, they can converse with data naturally and understand insights better. Self-service analytics removes technical barriers by offering natural language search, conversational user interfaces, and decision intelligence, thus making users self-reliant in adopting analytics.
6. Choose a self-service data analytics platform that is right for your organization
When evaluating a self-service analytics platform, look for abilities such as easy data integrations, self-service capabilities, customizable AI and machine learning models, embeddable APIs, and natural language processing. Additionally, a simple and intuitive user interface that enables business users to interact with data naturally ensures that they can do their own analysis without learning new skills or depending on analysts. These abilities will offer good returns on your analytics investments.
Challenges for Self-Service Analytics
Self-service analytics platforms that overcome the following challenges can benefit organizations to a great extent.
1. Improving insight discovery experience
Most people, especially non-technical business users, are overwhelmed with the amount of data and the technical aspects that are usually associated with analytics. This makes them hesitant to adopt analytics and forces them to fall back on their guess, intuition, or gut feeling. The main challenge for self-service analytics is to break these technological barriers, simplify analytics, and make the insight discovery experience conversational and intuitive for users. User-friendly interface, natural language search, and embedded search capabilities help improve the insight discovery experience.
2. Improving data literacy
Business decision makers are usually pressed for time, and often find themselves in critical situations that require immediate action. Drawing conclusions from a report, interpreting a chart, focusing on key data points in a cluster, or identifying an anomaly requires high data literacy skills that all users may not have. Self-service analytics can address this challenge by improving the presentation and summarization of insights. While interactive visualizations with drill-down capabilities, customized charts, text summaries, and audio-visual data stories can improve understanding and interpretation of business events, personalized business headlines can cut through the data noise to deliver what matters most to users.
3. Ensuring data access on need basis
It’s true that self-service analytics democratizes data so that everyone can access data to search and get answers. However, regulating this access is crucial to maintain data integrity and prevent its misuse. Self-service analytics can overcome this challenge by offering robust data governance policies that can be created and updated easily. Application of access policies at granular data levels, right from what users can search to how much they can share within teams, can help organizations maintain compliance and control over data.
How Self-Service Analytics Impacts Decision-Making
Self-service analytics encourages business decision makers to become not only self-reliant but also data-driven. According to PwC research, highly data-driven organizations are three times more likely to report significant improvement in decision-making. High-impact decisions such as where to invest profits, which channel to select for maximum reach, when to launch a new product, or which new region to venture into require a thorough study of the situation, a detailed examination of facts and numbers, comparison of current and past events, and correlation with influencing factors. Self-service analytics guides decision makers to do all of such thorough analysis effortlessly in a shorter amount of time.
Self-service analytics also creates a data-driven culture across the organization. Instead of relying on guesswork, intuition, or hearsay, users can base their decision on insights, since insights are readily available through self-service analytics. This also leads to improvement in the quality of decisions, ensures better outcomes, and minimizes the chances of failure. This way, self-service analytics helps organizations reap the benefits of data-driven decision-making.
Empower Decision Makers with MachEye's Self-Service Analytics
Self-service analytics makes everyone data-driven, independent, productive, and confident in their decisions and work. Empower your decision makers, irrespective of their skill level and functional areas, to search, analyze, and interact with complex data in seconds with MachEye, powered by LLMs. With its intuitive user interface, intelligent search, and interactive visualizations, MachEye’s self-service analytics platform leverages the power of AI, ML, NLP and data storytelling to enable quick and easy conversations with data. MachEye makes insights actionable and consumable, and helps users understand the What-Why-How of business events.